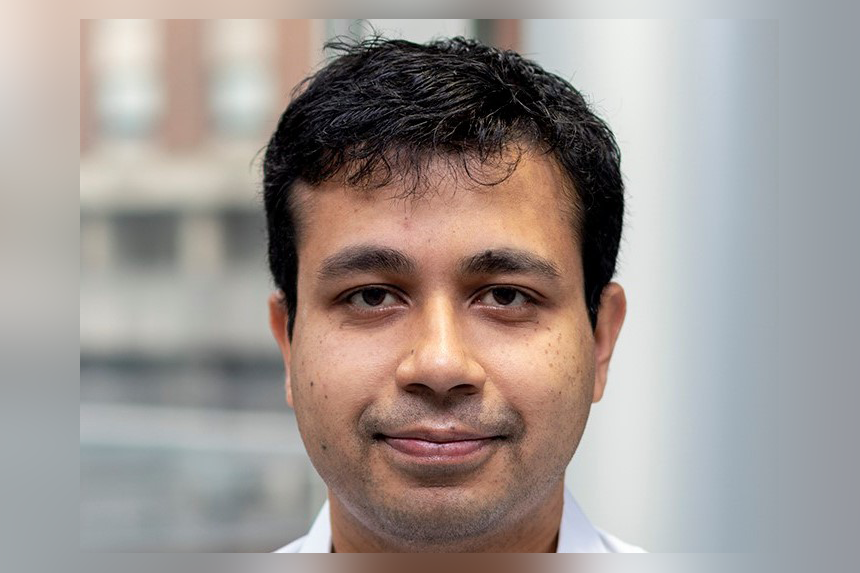
Statistical Sciences ARES: Jishnu Das
Join us at the Data Science Applied Research and Education Seminar (ARES) with:
Jishnu Das
Assistant Professor, Center for Systems Immunology
Departments of Immunology and Computational & Systems Biology
University of Pittsburgh
Free Hybrid Event | Registration Required
Talk Title:
Using Interpretable Machine Learning and Network Systems Approaches to Uncover Mechanisms Underlying Pathophysiology of Immune Disorders
Abstract:
Modern multi-omic technologies can generate deep multi-scale profiles. However, differences in data modalities, multicollinearity of the data, and large numbers of irrelevant features make multi-omic integration extremely challenging. Existing predictive machine learning approaches simply identify correlative biomarkers which may have no causal link to the outcome of interest. Here we present Significant Latent factor Interaction Discovery and Exploration (SLIDE), a novel interpretable machine learning technique that can integrate high-dimensional multi-omic datasets and identify putative causal latent factors underlying the response/outcome of interest. SLIDE makes no assumptions regarding data-generating mechanisms, comes with theoretical guarantees regarding identifiability and corresponding inference, incorporates non-linear relationships, and captures epistasis between these latent factors. SLIDE outperforms/performs at least as well as state-of-the-art approaches in terms of prediction, and provides inference beyond prediction. Several of the novel inferences offered by SLIDE were also borne out in targeted validation experiments.
Using SLIDE, we first sought to uncover altered cell-type-specific regulatory mechanisms underlying diffuse systemic sclerosis (SSc) pathogenesis. Using scRNA-seq profiles from skin biopsies of SSc subjects, SLIDE was able to accurately predict disease severity, and outperformed/performed as well as several benchmarks including LASSO, principal components regression (PCR), partial least squares regression (PLSR) and PHATE regression. Further, the interacting latent factors uncovered by SLIDE pointed to three distinct mechanisms. The first encompassed altered transcriptomic states in myeloid cells and fibroblasts, a well-elucidated basis of SSc disease severity. The second included an unexplored keratinocyte-centric signature, which we validated using protein staining. Finally, SLIDE uncovered a novel mechanism involving an interaction between the altered transcriptomic states in myeloid cells and fibroblasts with HLA signaling in macrophages. This mechanism has strong support in recent genetic association analyses, and demonstrates the power of SLIDE in unveiling novel biological mechanisms.
Next, we used SLIDE to elucidate latent factors underlying differences in clonal expansion of CD4 T cells in T1D. Using paired scRNA-seq and TCR-seq data on islet-derived cells in a non-obese diabetic (NOD) mouse model, we labeled cells based on their clonal expansion. SLIDE was able to accurately predict extent of clonal expansion, and outperformed/performed as well as the prior benchmarks. The latent factors uncovered by SLIDE included well-known markers of T cell exhaustion and clonal expansion including Lag3 and Cd200, that standard differential expression (DE) analyses would have picked up. However, it also picked up several novel genes, including Trbv10 and Trbv13.2 (TCR beta variable genes), that standard DE analyses would not have identified. We were able to hone in on functional roles for several of these genes.
We also applied SLIDE in a range of other contexts including the study of immune cell partitioning by spatial localization from spatial transcriptomic data. SLIDE consistently outperformed benchmarks, and provided novel inference not afforded by other approaches. Thus, SLIDE is a novel versatile interpretable machine learning framework for biological discovery from modern multi-omic datasets.
Speaker Profile:
I am a computational systems immunologist. I received my PhD from Cornell University in Computational Biology in 2016. After a short postdoc at MIT/the Ragon Institute of MGH, MIT, and Harvard, I started my systems immunology lab at the University of Pittsburgh. I have interdisciplinary expertise (47 published papers, primary contributor in 27) in using networks systems (examples – Wang*, Wei*, Thijssen*, Das* et al Nature Biotechnology 2012; Das et al Science Signaling 2013; Wei*, Das* et al PLoS Genetics 2014; Das et al Human Mutation 2014; Vo*, Das* et al Cell 2016; Fragoza, Das* et al Nature Communications 2019; Ningappa … Das^ Cell Reports Medicine 2022) and machine learning approaches (examples – Ackerman, Das et al Nature Medicine 2018; Suscovich*, Fallon*, Das* et al Science Translational Medicine 2020; Das et al PLoS Pathogens 2020; Das et al Med (Cell Press) 2021; Bing … Das^ Patterns (Cell Press) 2022; Pedireddy … Das^ Cell Reports 2022; *=co-first, ^=corresponding author) to analyze multi-omic datasets. My past work has analyzed Mendelian mutations in the context of three-dimensional protein networks, to understand molecular mechanisms of corresponding disorders, taking into account evolutionary dynamics. I have also used statistical and machine-learning approaches on high-dimensional molecular datasets to elucidate robust correlates of vaccine-mediated and natural immunity in HIV, malaria, and tuberculosis. Currently, we use machine learning and network approaches to integrate multi-modal datasets and identify molecular phenotypes in a range of immune disorders. My lab is currently supported by 13 NIH, DoD, and other agency grants, including by 3 R01/R01-equivalent grants on which I am a PI/MPI.
The event is finished.
Local Time
- Timezone: America/New_York
- Date: Nov 28 2022
- Time: 3:30 pm - 4:30 pm
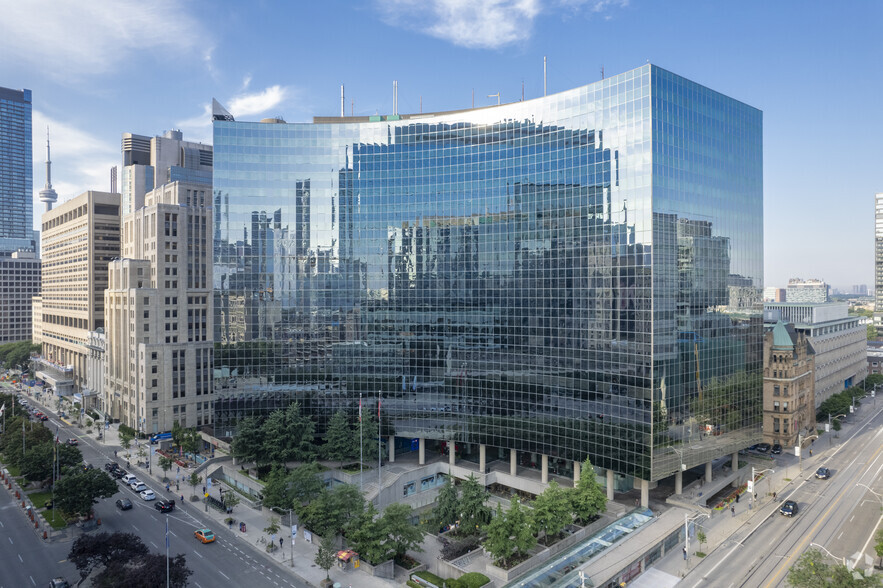
Location
Labels
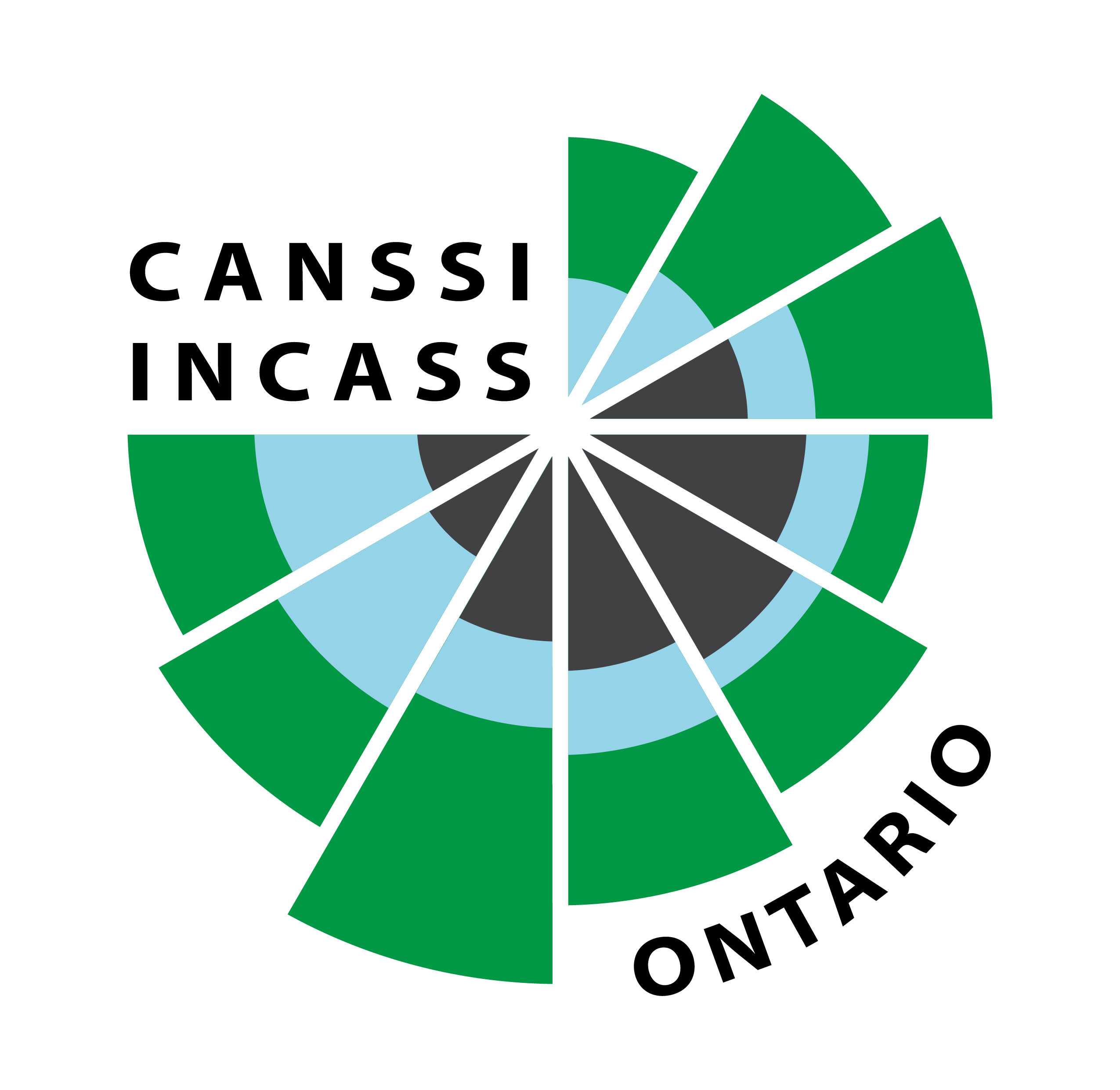
Organizer
CANSSI Ontario
Website
https://canssiontario.utoronto.caModerator
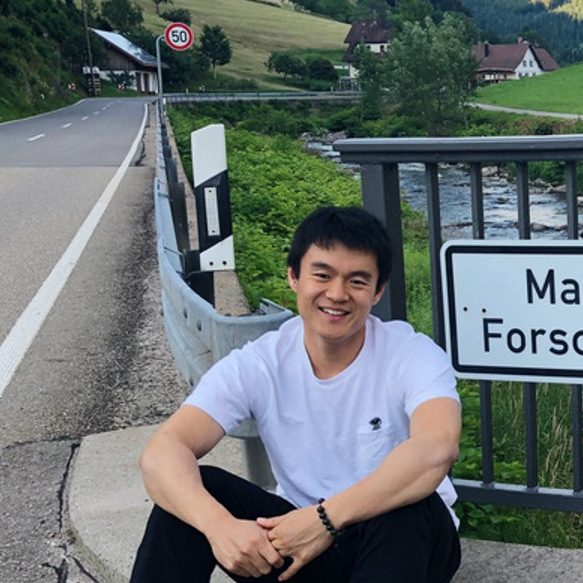
Mike Bing
Website
https://www.artsci.utoronto.ca/about/glance/new-faculty/faculty-profile-xin-mike-bingAssistant professor, Department of Statistical Sciences, University of Toronto