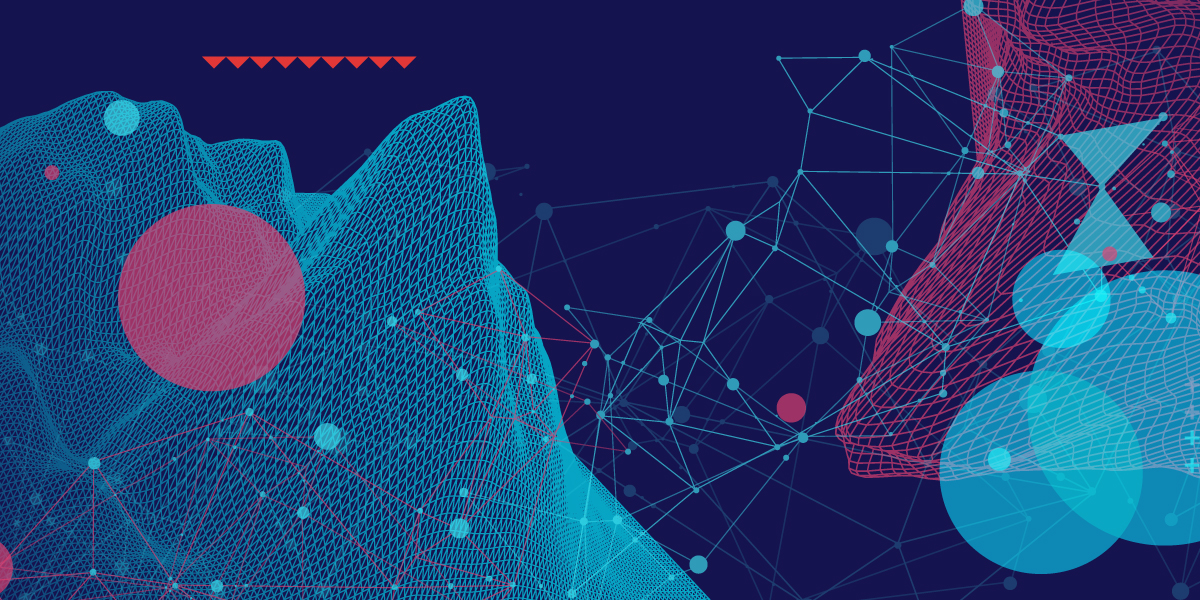
CANSSI Ontario Research Day 2022
This is a free, hybrid event that welcomes attendees both online (via Zoom) and in person (at Chestnut Conference Centre, 89 Chestnut St, Toronto, ON).
Overview
The CANSSI Ontario Research Day will showcase the work and discoveries by the data sciences and statistics community in Ontario. This one-day event draws participants from many Ontario universities, public, not-for-profit, and the research sector for a full day of data and discoveries.
2022 Theme
There is much debate among practitioners and scholars about what data science is. Join us on September 29, 2022, where you will hear some of the top experts in the field engage in informed, insightful discussions as they unravel the definitions of data science.
Registration
Registration is free.
Virtual webinar link:
https://us06web.zoom.us/j/81789056933?pwd=ODBTVkFseDFxbkJWZGZGVHR1bGEzUT09
Passcode: 122701
Lunch, as well as drinks and snacks during the morning and afternoon breaks, will be provided.
Seating is available on a first come, first served basis, subject to venue capacity
Poster
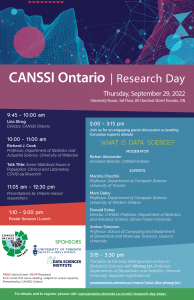
Vote for best CANSSI Ontario Trainee presentation here:
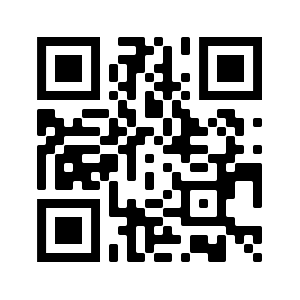
Sponsors:

Hourly Schedule
September 29, 2022
- 9:45 am - 10:00 am
- Welcoming Remarks
-
Speakers:
Lisa Strug
- 10:00 am - 11:00 am
- Some Statistical Issues in Population, Clinical and Laboratory COVID-19 Research
- The COVID-19 pandemic has stimulated intensive interdisciplinary collaborations aiming to advance understanding of the population dynamics of infection, develop vaccines, and identify effective therapeutic interventions. This talk will describe three public health, clinical and laboratory research projects in COVID-19 research with an emphasis on the statistical challenges and methodology. The projects will be presented in the order they arose over the course of the pandemic. Specific topics include reporting delay adjustments to provincial and national infection rates, rapid design and cost-effective execution of a clinical trial assessing the therapeutic effect of convalescent plasma, and evaluating the vaccine response to individuals with autoimmune disease. Understanding the data acquisition and reporting processes is shown to be critically important, highlighting the need for careful planning as well as a good public health infrastructure for population research.
-
Speakers:
Richard Cook
- 11:00 am - 11:05 am
- Break
- 11:05 am - 1:10 pm
- Presentations by Ontario-based Researchers
- Gengming He, Division of Biostatistics, Dalla Lana School of Public Health, University of Toronto
Develop novel statistical methods for analyzing long-read sequencing data to investigate the genetic mechanism of cystic fibrosis
David Li, Department of Statistical Sciences, University of Toronto.
A Poisson Cluster Process with Cluster-Dependent Marking for Detecting Ultra-Diffuse Galaxies
Boxi Lin, Division of Biostatistics, Dalla Lana School of Public Health, University of TorontoSex-stratified vs. sex-combined analysis in the presence of genetic effect heterogeneity.
YuChung Lin, Division of Biostatistics, Dalla Lana School of Public Health, University of Toronto
Incorporating Functional Annotations in Polygenic Risk Scores Improves Generalizability to Cross-Ethnic Populations
Samar Salah Mohamedahmed, Center for Addiction and Mental Health (CAMH), Pharmacogenetics Research Clinic
Genetic and Polygenic Risk Analysis of Antidepressant Response and Cognitive Domains in Late-Life Depression
Alina Selega, Lunenfeld-Tanenbaum Research Institute
Multi-objective Bayesian Optimization with Heuristic Objec- tives for Biomedical and Molecular Data Analysis Workflows
Divya Sharma, Division of Biostatistics, Dalla Lana School of Public Health, University of Toronto
Hybrid CNN-LSTM model for disease prediction using longitudinal microbial data
Teresa Tsui, Sunnybrook Research Institute
Accounting for uncertainty in health utilities to inform cancer drug funding decisions
Nicholas, Waglechner, Lunenfeld-Tanenbaum Research Institute, Sinai Health in Toronto
Genomic Epidemiology of Mycobacterium abscessus in a Canadian Cystic Fibrosis Centre
Changchang Xu, Division of Biostatistics, Dalla Lana School of Public Health, University of Toronto
Penalized maximum likelihood inference of mixture cure model under multiple imputation
Jingxiong Xu, Lunenfeld-Tanenbaum Research Institute
A Novel Gene-Based Test for Sequencing Studies Based on a Bayesian Variable Selection of Rare Variants
Ziang Zhang, Department of Statistical Sciences, University of Toronto.
An indirect test of gene-environment interaction for binary trait
Lehang Zhong, Division of Biostatistics, Dalla Lana School of Public Health, University of Toronto.
RoPE: a robust profile likelihood method for differential gene expression analysis
Junhao Zhu, Department of Statistical Sciences, University of Toronto.
LLOT in Reconstruction of Spatial Expression
-
Speakers:
Alina Selega, Boxi Lin, Changchang Xu, Dayi (David) Li, Divya Sharma, Gengming He, Jingxiong Xu, Junhao Zhu, Lehang Zhong, Samar Salah Mohamedahmed Elsheikh, Teresa Tsui, YuChung Lin, Ziang Zhang
- 1:10 - 2:00 pm
- Poster Session | Lunch
- 2:00 - 3:15 pm
- Panel Discussion: What is Data Science?
- Moderated by:
Rohan Alexander, Assistant Director, CANSSI Ontario; Assistant Professor, Faculty of information and Department of Statistical Sciences, University of Toronto
Panellists:
Marsha Chechik, Professor, Department of Computer Science, University of Toronto
Mark Daley, Chief Digital Officer, Professor, Department of Computer Science, University of Western Ontario
Donald Estep, Director, CANSSI; Professor, Department of Statistics and Actuarial Science, Simon Fraser University
Amber Simpson, Professor, School of Computing and Department of Biomedical and Molecular Sciences, Queen’s University
- 3:15 - 3:30 pm
- Transition to the Distinguished Lecture Series in Statistical Sciences (DLSS) with Dr. Xihong Lin
- Registration for the DLSS is separate and available here
-
Speakers:
Xihong Lin
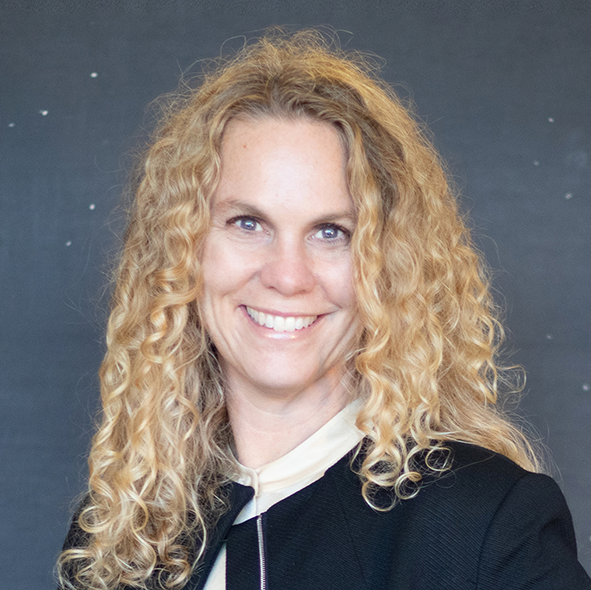
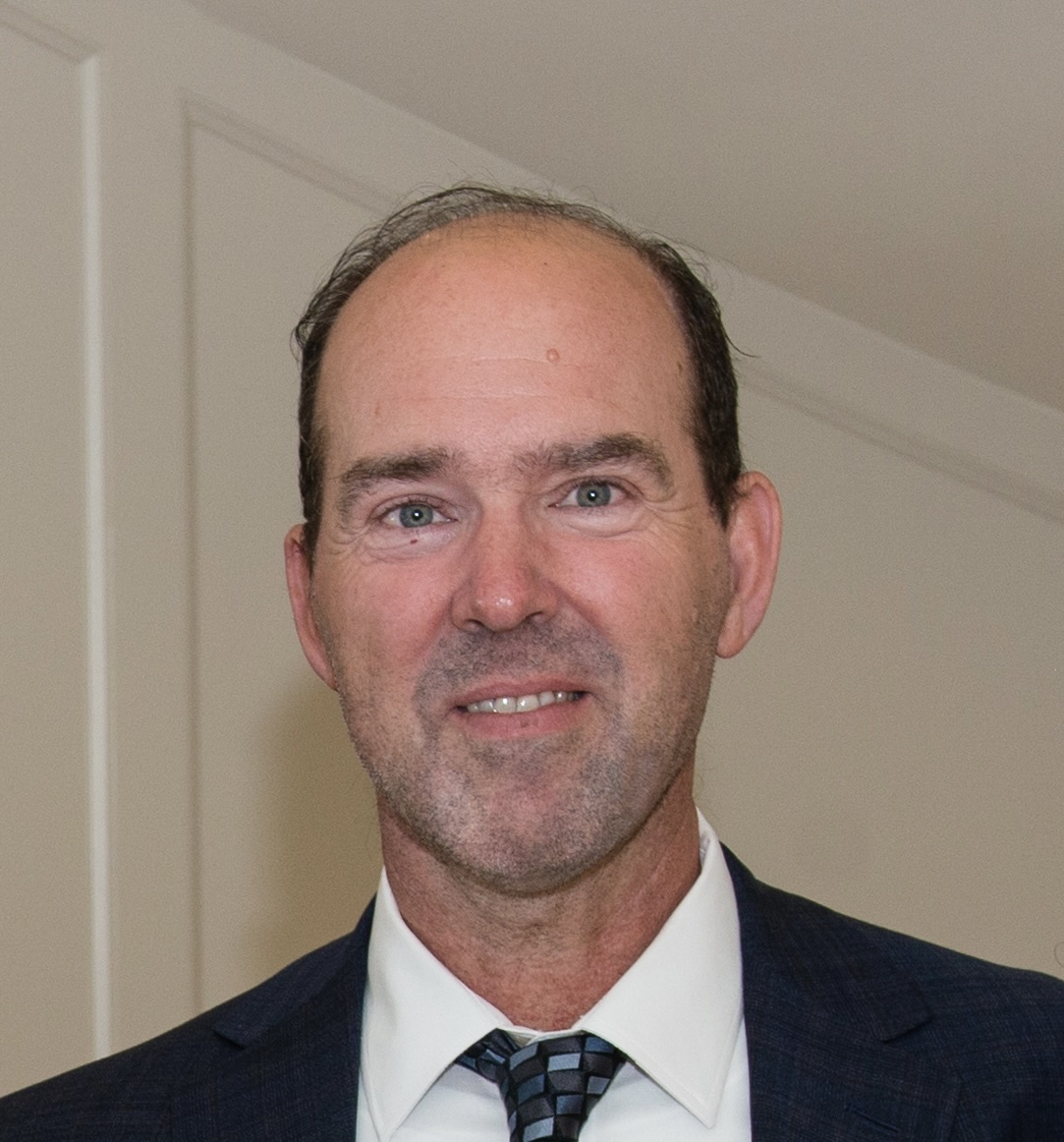
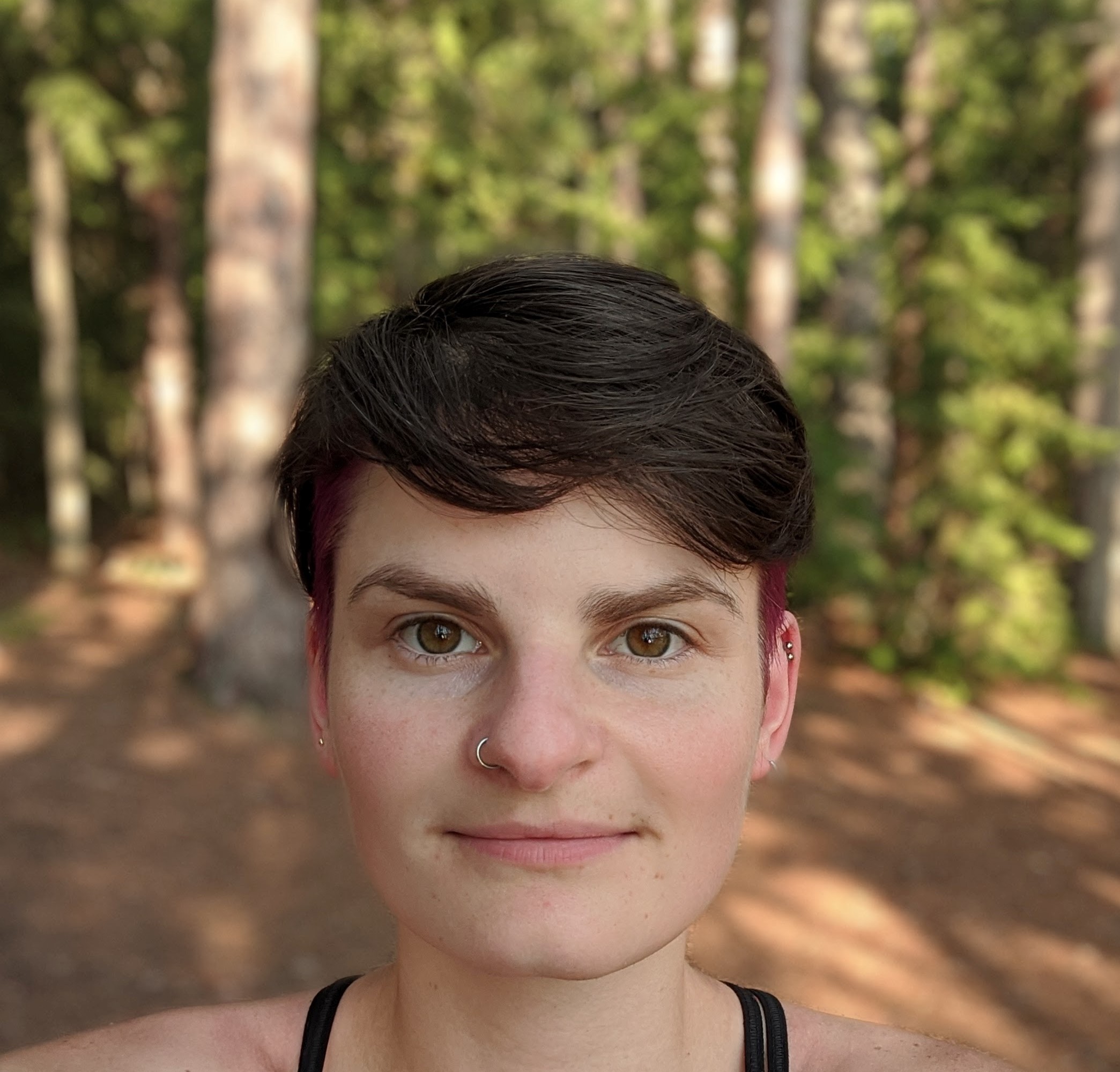
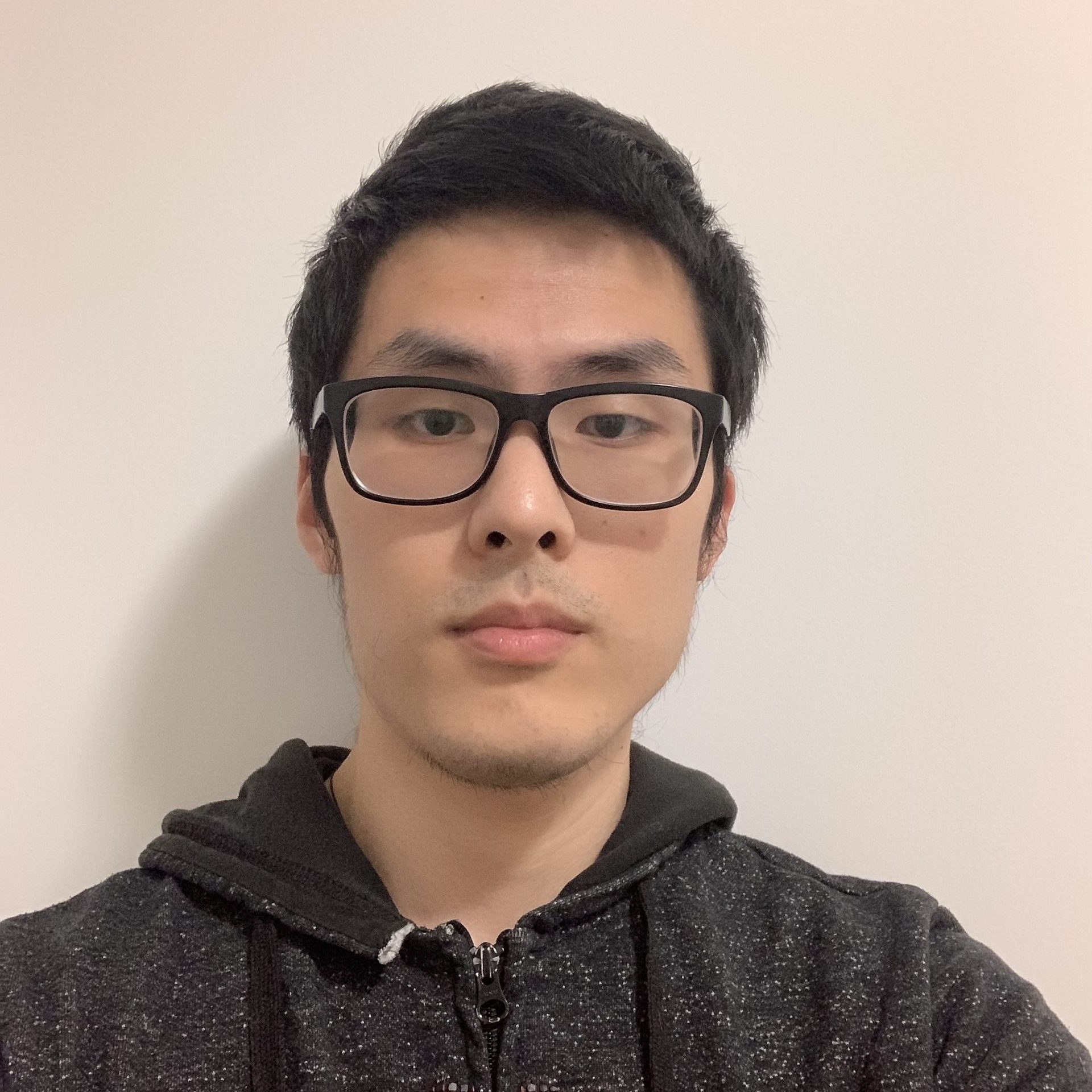
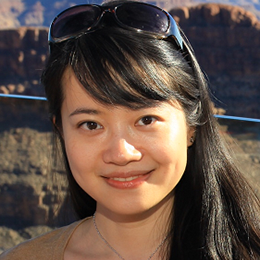
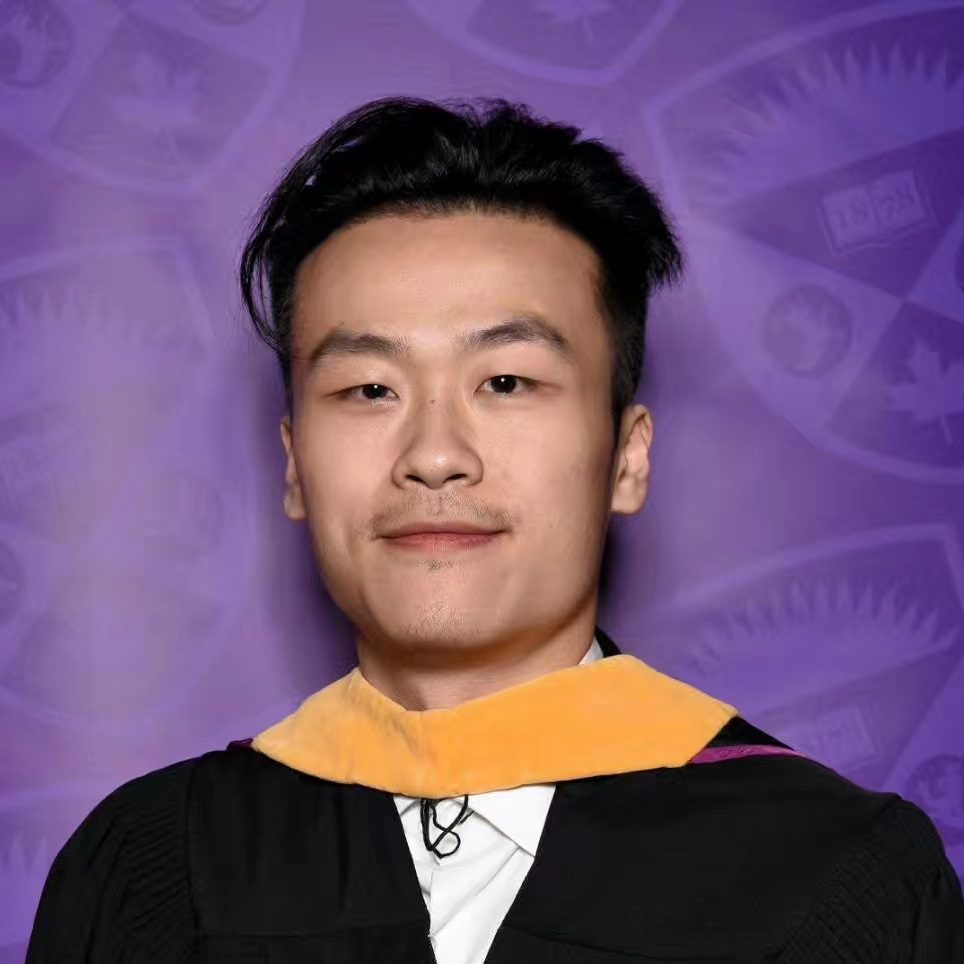
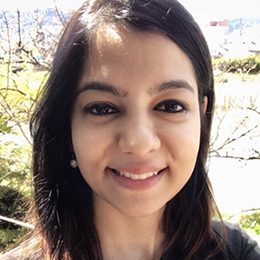
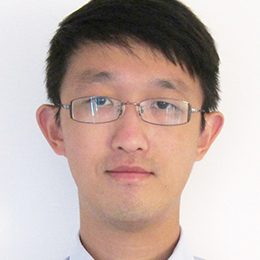
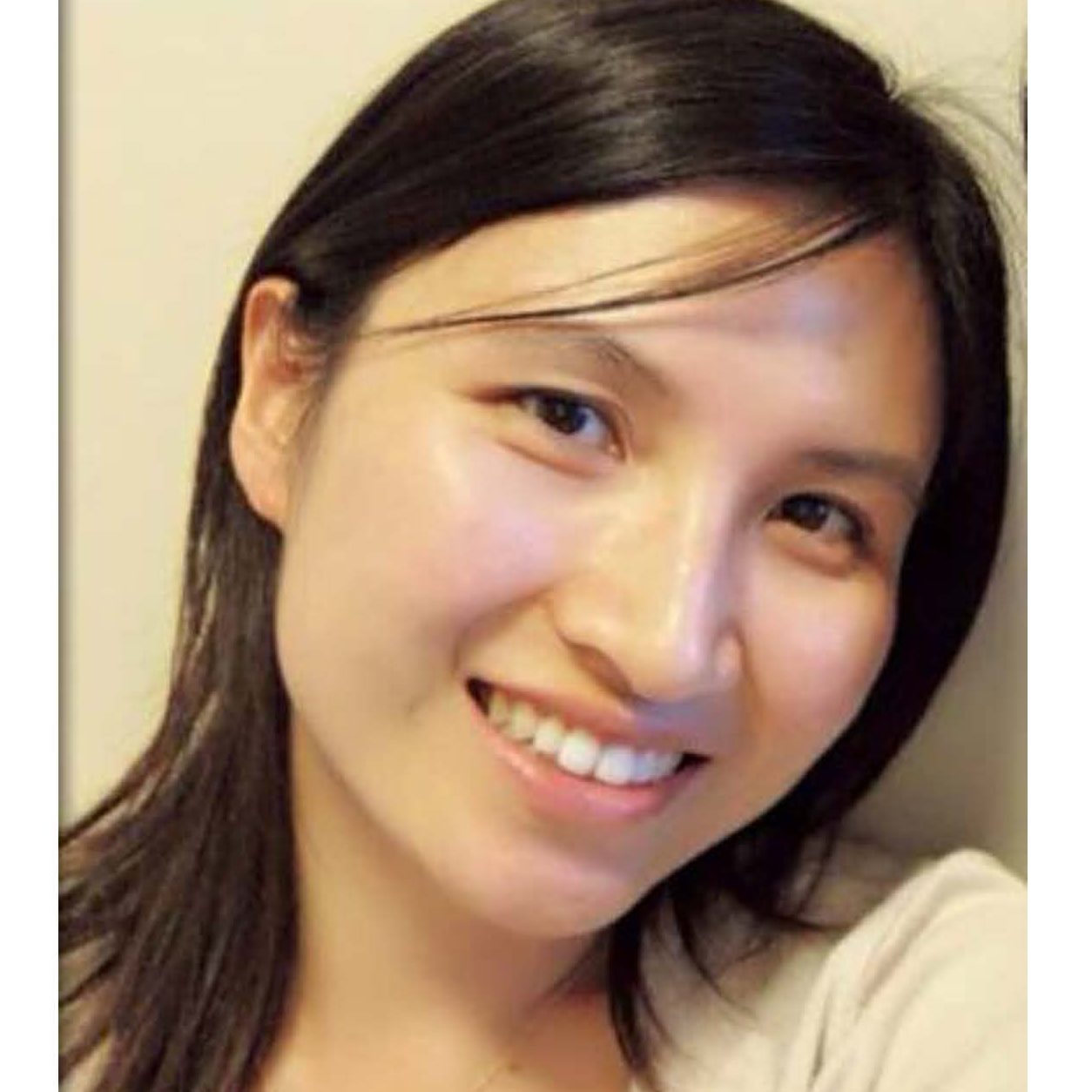
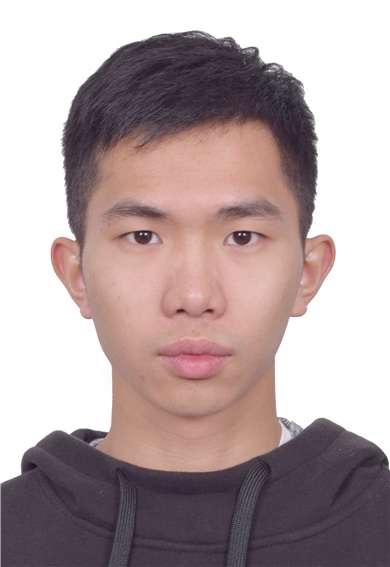
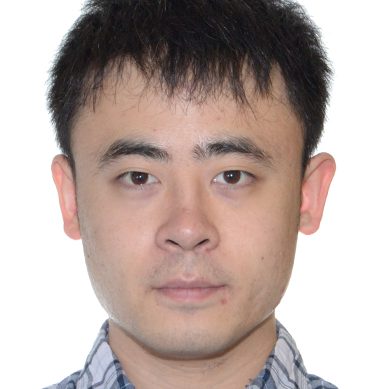
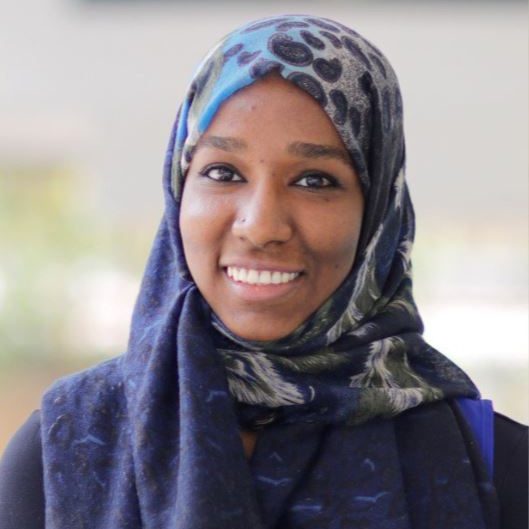
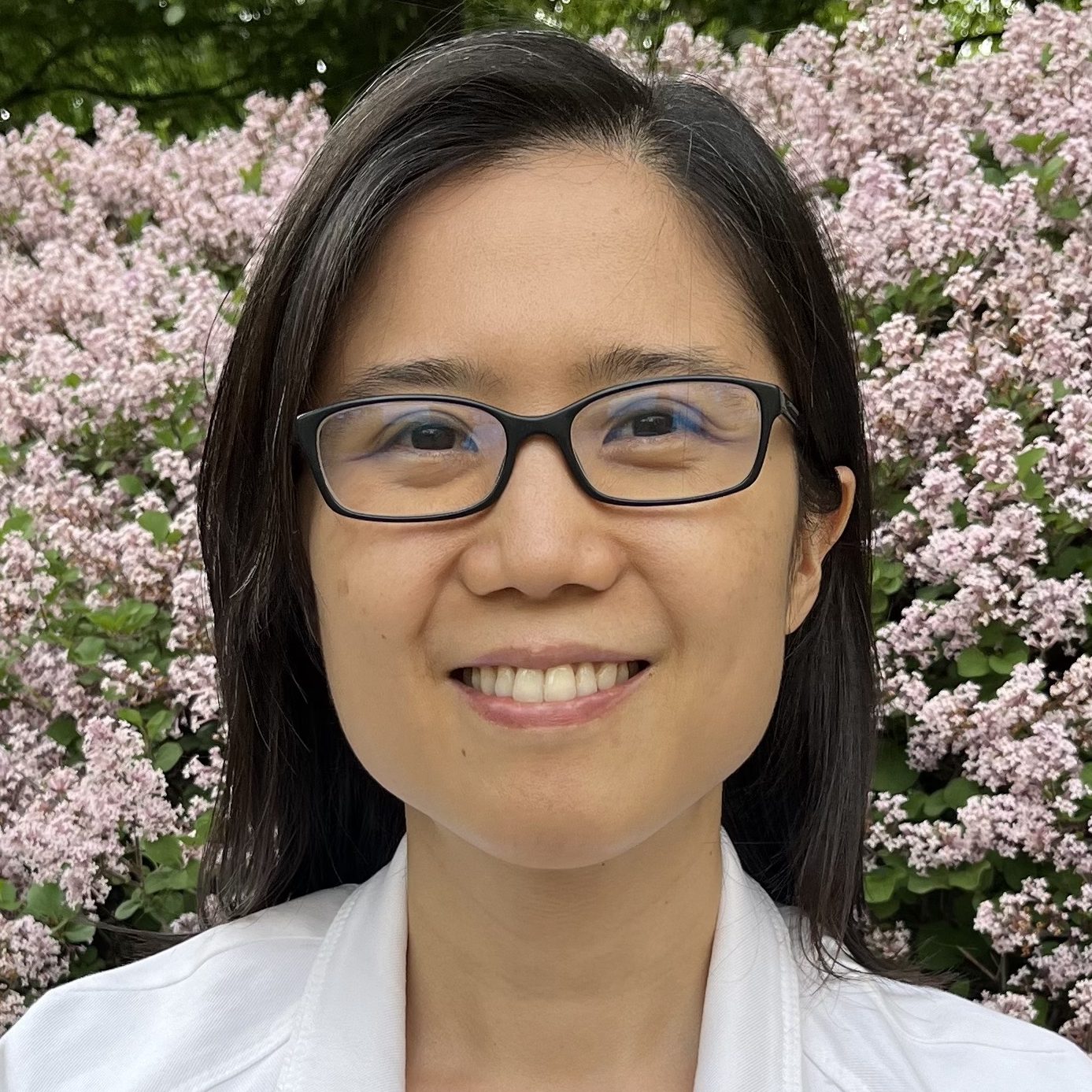
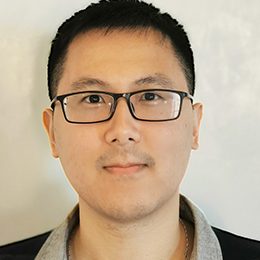
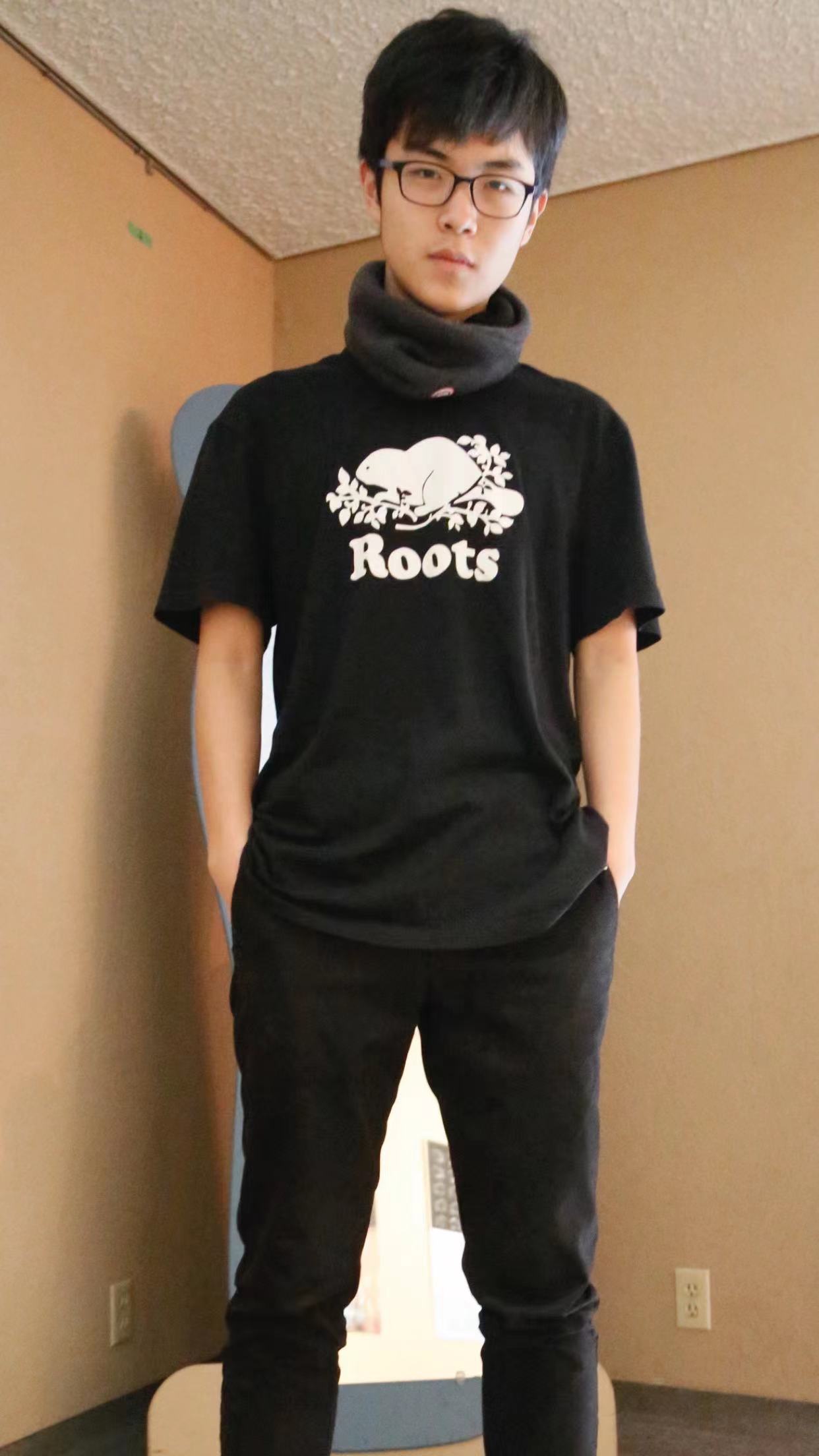
The event is finished.
Local Time
- Timezone: America/New_York
- Date: Sep 29 2022
- Time: 9:45 am - 5:30 pm
Location
Location 2
Speakers
-
Richard CookUniversity Professor and Mathematics Faculty Research Chair, Department of Statistical and Actuarial Sciences, Faculty of Mathematics; University Waterloo
Richard Cook is a Professor in the Department of Statistics and Actuarial Science, Faculty of Mathematics Research Chair, and University Professor at the University of Waterloo. He holds a cross-appointment in the School of Public Health (UW) and a part-time appointment in the Faculty of Health Science at McMaster University. His research interests include the analysis of life history data, the design and analysis of clinical and epidemiological studies, and statistical methods for the analysis of incomplete data. He has published extensively in these areas and written two books with Jerry Lawless. He is also deeply engaged in collaborative research with other scientists working in transfusion medicine, immunology, and cancer, and consults widely with industry and government organizations. In 2018 he was awarded the Gold Medal of the Statistical Society of Canada and in 2021 he was named a Fellow of the Royal Society of Canada.