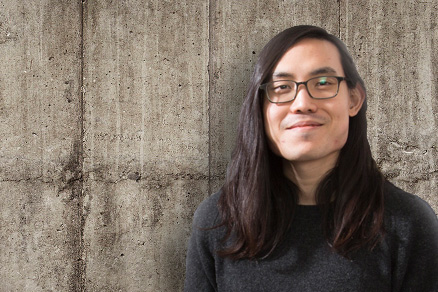
Data Sciences Speaker Series at UofT: Dustin Tran
Join us for the next installment of the Data Science Speaker Series at U of T with
Dr. Dustin Tran
Research Scientist
Google Brain
Free Event | Registration Required
Talk Title: Reliability for Deep Learning
Abstract: Deep learning models are bad at signalling failure: They can make predictions with high confidence, and this is problematic in real-world applications such as healthcare, self-driving cars, and natural language systems, where there are considerable safety implications, or where there are discrepancies between the training data and data that the model makes predictions on. There is a pressing need both for understanding when models should not make predictions and improving model robustness to natural changes in the data. We’ll give an overview of this problem setting. We also highlight promising avenues from recent work, including methods which average over multiple neural network predictions such as Bayesian neural nets and ensembles; as well as the use of large pretrained models.
Speaker Profile: Dustin is a research scientist at Google Brain. His research contributions examine the intersection of probability and deep learning, particularly in the areas of probabilistic programming, variational inference, giant models, and Bayesian neural networks. He completed his Ph.D. at Columbia under David Blei. He’s received awards such as the John M. Chambers Statistical Software award and the Google Ph.D. Fellowship in Machine Learning. He served as Area Chair at NeurIPS, ICML, ICLR, IJCAI, and AISTATS and organized “Approximate Inference” and “Uncertainty & Robustness” workshops at NeurIPS and UAI.
The event is finished.
Local Time
- Timezone: America/New_York
- Date: Apr 25 2022
- Time: 5:15 pm - 6:15 pm
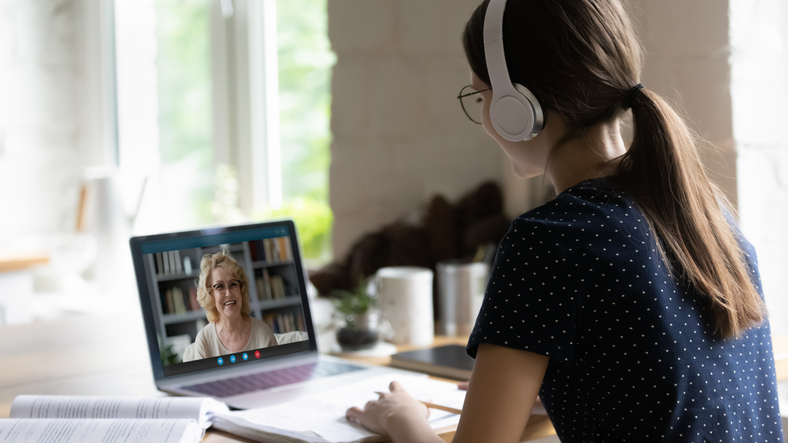