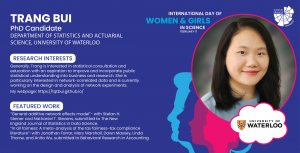
2023 International Day of Women and Girls In Science: Trang Bui
Trang Bui, PhD Candidate Department of Statistics and Actuarial Science,
Department of Statistics and Actuarial Science, University of Waterloo
Generally, Trang is interested in statistical consultation and education with an aspiration to improve and incorporate public statistical understanding into business and research. She is particularly interested in network-correlated data and is currently working on the design and analysis of network experiments.
Professors Stefan H. Steiner and Nathaniel T. Stevens
As a woman, I have always been self-conscious about the notion of time. I often feel intimidated and discouraged to continue my study as time passes and more responsibility comes. This has put me into depression. Gradually, I came to realize that I should stop worrying about the future and focus on what I’m currently doing. I should divide my problems into smaller pieces and tackle them one by one. This way I will feel less stressed and gain more confidence as I progress. I also realize that it is hard to figure things out on my own. I should be more open to communication and seek help and advice from other people when I am in need. I’m glad and grateful to know that there are many people out there willing to talk and help me when I reach out to them.
Statistics is the study of data through the lens of mathematics. Traditionally, statistics help us infer characteristics of the population even with a small sample. Nowadays, data has become increasingly available, and the problem evolves from small data to big data, where data sanity, data collecting process, and data integration are among the main issues. In addition, machine learning techniques, although very powerful, but also become more sophisticated with each model containing millions to billions of parameters trained in blackboxes. Therefore, I think another important challenge for future statistical research is to develop methods to correctly understand and interpret these models.
Trang Bui, PhD Candidate Department of Statistics and Actuarial Science,
Maryam Y. Onifade, PhD Candidate Department of Mathematics and Statistics,
Emma Kroell, PhD Candidate Department of Statistical Sciences, University of
Anita Shahrokhian, PhD Candidate Department of Mathematics and Statistics, Queen’s University
On December 22, 2015, the United Nations General Assembly established
CANSSI Ontario
10th Floor, Suite 10072
700 University Avenue
Toronto, ON M5G 1Z5